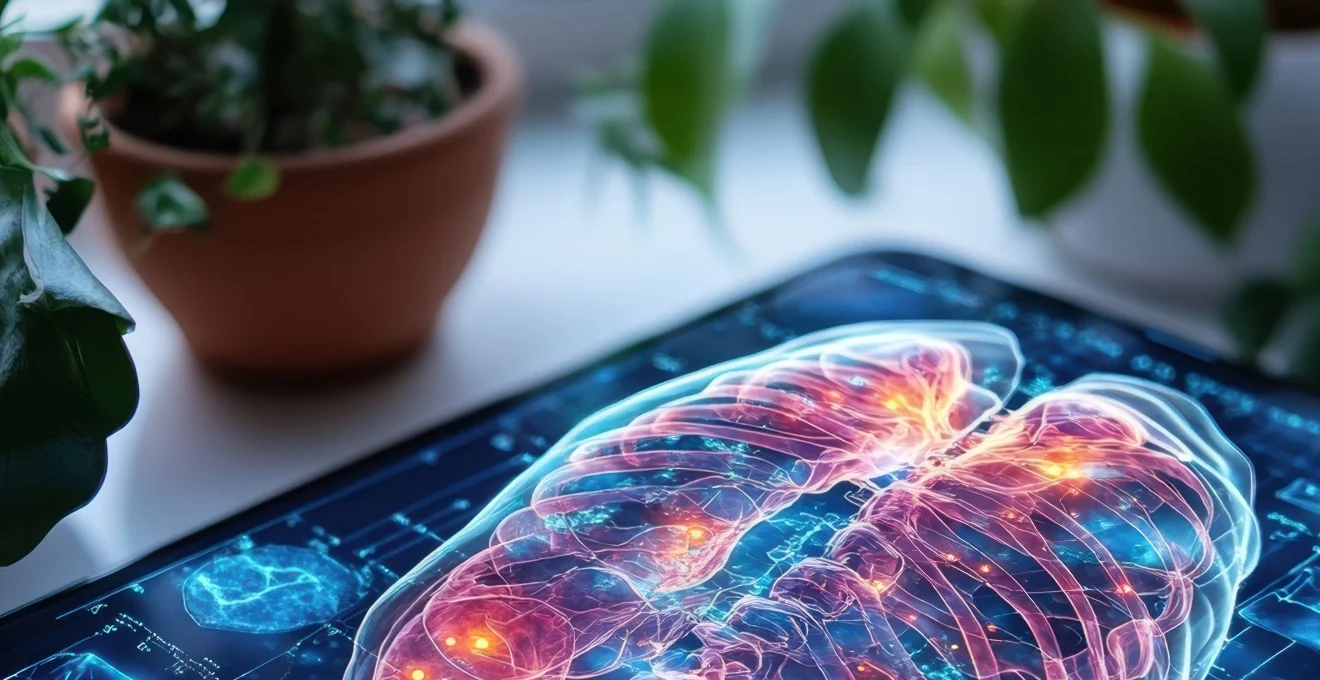
The convergence of artificial intelligence (AI) and healthcare is ushering in a new era of medical strategy. As data becomes increasingly central to medical decision-making, AI-enhanced analytics are transforming how healthcare professionals diagnose, treat, and manage patient care. This revolutionary approach is not only improving patient outcomes but also streamlining healthcare operations and reducing costs.
Ai-driven predictive analytics in healthcare Decision-Making
Predictive analytics powered by AI are revolutionizing healthcare decision-making processes. By analyzing vast amounts of patient data, including medical histories, genetic information, and lifestyle factors, AI algorithms can predict potential health risks and outcomes with remarkable accuracy. This capability allows healthcare providers to intervene proactively, potentially preventing diseases before they manifest or progress.
One of the most significant applications of AI-driven predictive analytics is in population health management. Healthcare organizations can now identify high-risk patients within a population and implement targeted interventions. For instance, AI models can predict which patients are most likely to develop chronic conditions like diabetes or heart disease, enabling early intervention and personalized prevention strategies.
Moreover, predictive analytics are enhancing clinical decision support systems. These AI-powered tools can assist physicians in making more informed decisions by providing real-time insights based on the latest medical research and patient data. As a result, you can expect to see more personalized treatment plans that take into account your unique health profile and risk factors.
As these technologies mature, you can expect to see even more sophisticated AI applications in healthcare. From ultra-personalized treatment plans based on quantum-powered genetic analysis to blockchain-enabled health data marketplaces that fuel advanced AI research, the future of healthcare is undoubtedly intertwined with the continued evolution of AI and related technologies. Experts in the field of medical strategy and innovation, such as those at alcimed.com, are at the forefront of exploring these emerging trends and their potential impact on healthcare delivery and patient outcomes.
Machine learning algorithms for medical data interpretation
Machine learning, a subset of AI, is particularly adept at interpreting complex medical data. Various types of machine learning algorithms are being employed across different areas of healthcare, each with its unique strengths and applications.
Convolutional neural networks in medical imaging analysis
Convolutional Neural Networks (CNNs) have revolutionized medical imaging analysis. These sophisticated algorithms can detect subtle patterns in medical images that might be imperceptible to the human eye. CNNs are particularly effective in analyzing radiological images such as X-rays, CT scans, and MRIs.
For example, CNNs can identify early signs of lung cancer in chest X-rays or detect minute changes in brain structure that could indicate the onset of neurodegenerative diseases. The ability of these algorithms to process and analyze thousands of images quickly and accurately is transforming diagnostic radiology, potentially leading to earlier detection and improved patient outcomes.
Natural language processing for electronic health records
Natural Language Processing (NLP) is another critical machine learning technique that's making significant strides in healthcare. NLP algorithms can interpret and extract meaningful information from unstructured text data, such as clinical notes and electronic health records (EHRs).
By analyzing EHRs, NLP can help identify patterns in patient histories, track disease progression, and even predict potential health risks. This technology is particularly valuable in improving the efficiency of clinical documentation and enhancing the quality of patient care by ensuring that no critical information is overlooked.
Random forest models in patient risk stratification
Random Forest models, an ensemble learning method, are proving invaluable in patient risk stratification. These models can analyze multiple variables simultaneously to predict patient outcomes and stratify patients based on their risk levels.
In practice, Random Forest algorithms can help identify patients at high risk of hospital readmission, predict the likelihood of postoperative complications, or determine the risk of adverse drug reactions. By stratifying patients based on risk, healthcare providers can allocate resources more effectively and implement targeted interventions where they're needed most.
Reinforcement learning for treatment optimization
Reinforcement Learning (RL) is an exciting frontier in AI-enhanced healthcare. This machine learning technique is particularly useful in optimizing treatment plans over time. RL algorithms can learn from the outcomes of different treatment strategies and continuously refine their recommendations.
In fields like oncology, RL can help optimize chemotherapy dosing regimens, balancing the need for effective treatment with the minimization of side effects. As more data becomes available, these algorithms can adapt and improve their recommendations, potentially leading to more effective and personalized treatment strategies.
Integration of big data in clinical strategy development
The integration of big data in clinical strategy development is transforming how healthcare organizations approach patient care and resource allocation. Big data analytics, powered by AI, allow healthcare providers to gain insights from vast and diverse datasets, including clinical, financial, and operational data.
This integration enables healthcare organizations to develop more effective clinical strategies based on real-world evidence. By analyzing patterns across large patient populations, healthcare providers can identify best practices, optimize treatment protocols, and improve overall patient outcomes.
IBM watson's role in oncology treatment planning
IBM Watson for Oncology is a prime example of how AI and big data are being integrated into clinical strategy development. This AI-powered system analyzes patient medical records, research publications, and clinical guidelines to provide evidence-based treatment recommendations for cancer patients.
Watson's ability to process and interpret vast amounts of medical literature and patient data allows it to stay current with the latest advancements in oncology. As a result, oncologists can access up-to-date, evidence-based treatment options tailored to each patient's specific case, potentially improving treatment outcomes and patient care quality.
Google DeepMind's contribution to diagnostics
Google DeepMind has made significant contributions to AI-enhanced diagnostics, particularly in the field of ophthalmology. Their AI system has demonstrated the ability to analyze retinal scans and detect signs of eye diseases with accuracy comparable to human experts.
This technology has the potential to revolutionize eye care, especially in areas with limited access to specialist ophthalmologists. By integrating such AI systems into clinical workflows, healthcare providers can improve early detection rates and ensure timely interventions for sight-threatening conditions.
Amazon web services' healthcare analytics solutions
Amazon Web Services (AWS) offers a range of healthcare analytics solutions that leverage big data and AI to improve patient care and operational efficiency. These cloud-based services enable healthcare organizations to securely store, process, and analyze large volumes of healthcare data.
AWS's machine learning tools can help healthcare providers predict patient admission rates, optimize staffing levels, and identify potential cost-saving opportunities. By harnessing the power of cloud computing and AI, healthcare organizations can make data-driven decisions that improve both patient outcomes and operational efficiency.
Real-time patient monitoring and AI-Enhanced telemedicine
AI is playing a crucial role in advancing real-time patient monitoring and telemedicine capabilities. With the proliferation of wearable devices and Internet of Things (IoT) sensors, healthcare providers can now continuously monitor patients' vital signs and other health metrics remotely.
AI algorithms can analyze this continuous stream of data in real-time, alerting healthcare providers to any concerning changes or potential health risks. This capability is particularly valuable for managing chronic conditions and reducing hospital readmissions.
In the realm of telemedicine, AI is enhancing the quality and efficiency of remote consultations. AI-powered chatbots can conduct initial patient screenings, while natural language processing algorithms can assist in transcribing and analyzing telehealth conversations. These technologies not only improve the patient experience but also allow healthcare providers to serve a larger number of patients more effectively.
Ethical considerations and data privacy in AI-Powered healthcare
As AI becomes increasingly integrated into healthcare systems, ethical considerations and data privacy concerns are at the forefront of discussions among healthcare professionals, policymakers, and patients. While the potential benefits of AI in healthcare are immense, it's crucial to address these concerns to ensure responsible and equitable implementation.
HIPAA compliance in AI-Enhanced analytics systems
Ensuring HIPAA compliance in AI-enhanced analytics systems is paramount. Healthcare organizations must implement robust security measures to protect patient data, especially when using cloud-based AI solutions. This includes encryption of data both in transit and at rest, secure access controls, and regular security audits.
Moreover, AI systems must be designed with privacy in mind from the outset. This concept, known as "privacy by design," ensures that data protection is built into the core functionality of AI-enhanced analytics systems rather than being added as an afterthought.
Addressing bias in medical AI algorithms
Bias in AI algorithms is a significant concern in healthcare. If not carefully addressed, AI systems can perpetuate or even exacerbate existing healthcare disparities. For instance, if an AI algorithm is trained on data that underrepresents certain demographic groups, it may produce less accurate results for those populations.
To mitigate this risk, it's crucial to ensure diversity in the datasets used to train AI algorithms. Additionally, ongoing monitoring and validation of AI systems are necessary to detect and correct any biases that may emerge over time. Healthcare organizations must also prioritize diversity in their AI development teams to bring a range of perspectives to the design and implementation of these systems.
Patient consent and data ownership in the AI era
As AI systems rely on vast amounts of patient data, questions of consent and data ownership become increasingly complex. Patients must be fully informed about how their data will be used in AI systems and given the opportunity to provide informed consent.
Furthermore, the concept of data ownership in the context of AI-generated insights is an area of ongoing debate. Healthcare organizations and policymakers must work together to establish clear guidelines on who owns the insights generated by AI systems and how these insights can be used.
Regulatory frameworks: FDA's approach to AI in medical devices
The U.S. Food and Drug Administration (FDA) is actively developing regulatory frameworks to govern the use of AI in medical devices. The FDA's approach aims to balance the need for innovation with patient safety considerations.
One key aspect of the FDA's strategy is the concept of "adaptive" AI systems - those that can learn and change over time. The regulatory framework for such systems must be flexible enough to allow for continuous learning while ensuring ongoing safety and efficacy.
Future trends: quantum computing and blockchain in medical AI
Looking ahead, emerging technologies like quantum computing and blockchain are poised to further revolutionize AI in healthcare. Quantum computing has the potential to dramatically accelerate complex calculations, potentially leading to breakthroughs in drug discovery and personalized medicine.
Blockchain technology, on the other hand, could provide a secure and transparent way to manage health data, addressing many of the current concerns around data privacy and interoperability. By creating a decentralized and immutable record of health data transactions, blockchain could enable more secure and efficient sharing of health information across different healthcare providers and AI systems.